Welcome to AIAppBuilder
Discover insights, stories, and expertise from our community
Explore ArticlesA Word from Our Expert
"In today's rapidly evolving digital landscape, staying informed and adaptable is key to success. Our platform brings you cutting-edge insights and practical knowledge from industry leaders."
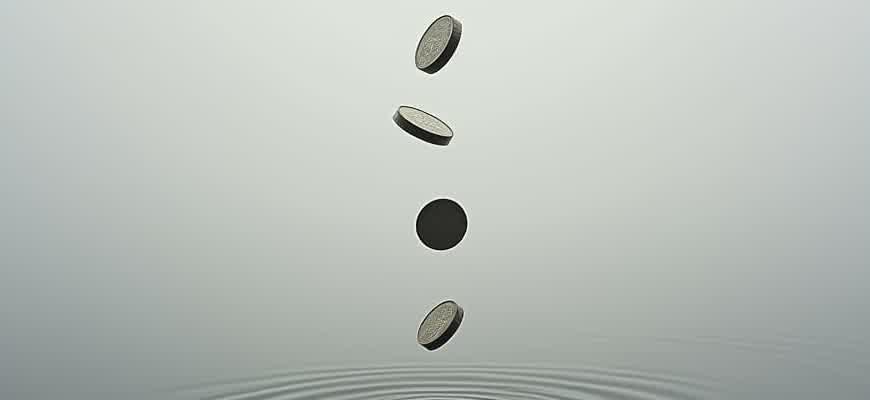
Featured Articles
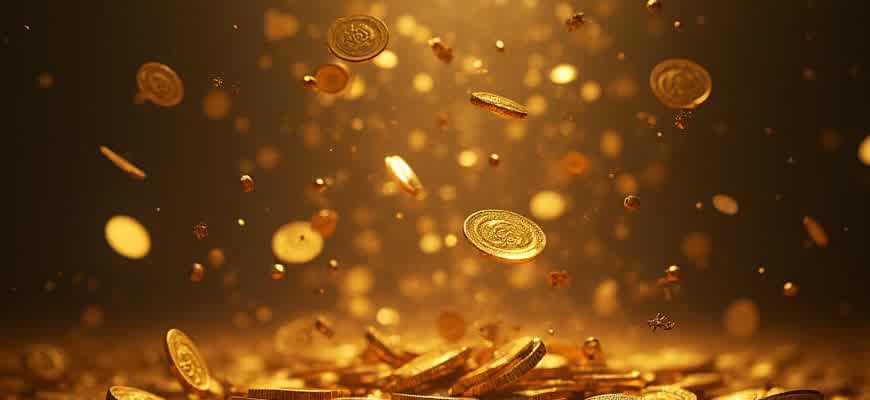
K Hovnanian Builder Reviews
K Hovnanian is a prominent name in residential construction, known for offering a variety of homes across the United States. As with any builder, cus...
Read More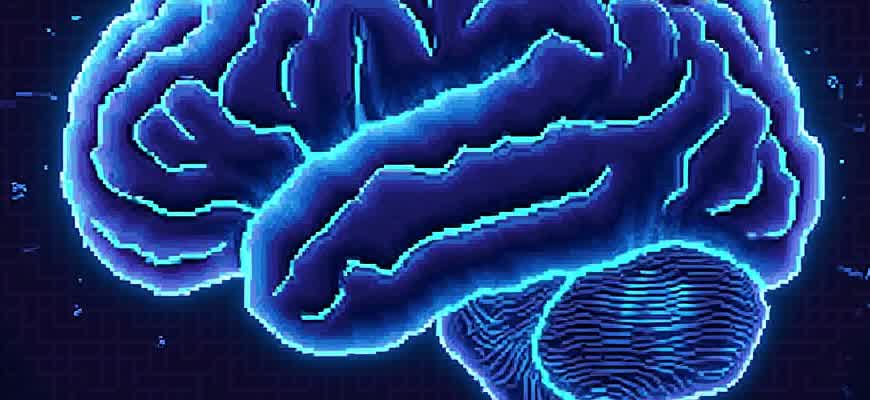
3d Collaboration Software
Modern industries demand advanced solutions for remote collaboration, and 3D collaboration software offers just that. These platforms allow multiple ...
Read More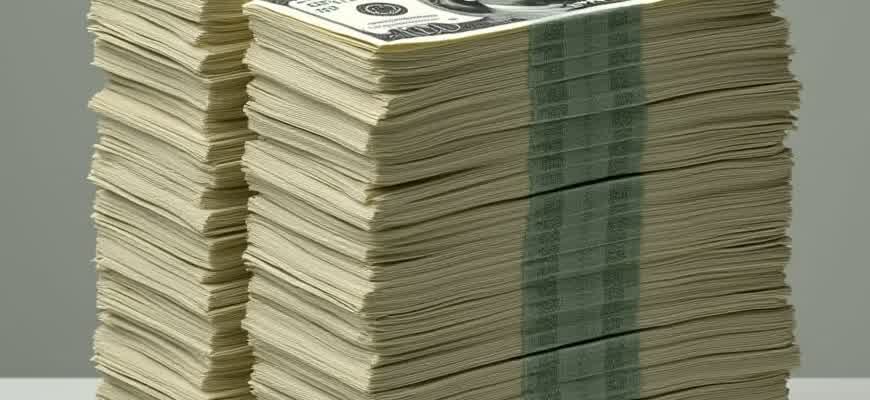
Zoho Creator Mobile App Development
Zoho Creator offers a robust platform for building custom mobile applications without extensive coding knowledge. This low-code tool enables business...
Read More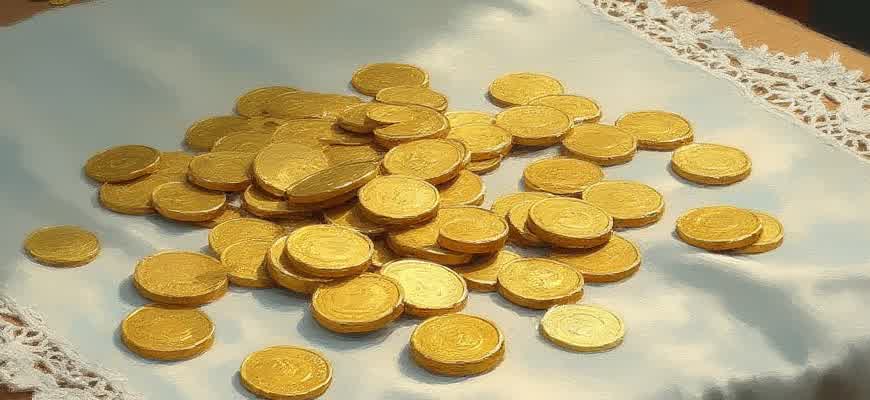
Which App Is Best for Designing
Designing has evolved with technology, and the choice of tools can significantly affect the outcome of any project. Whether you're a professional des...
Read More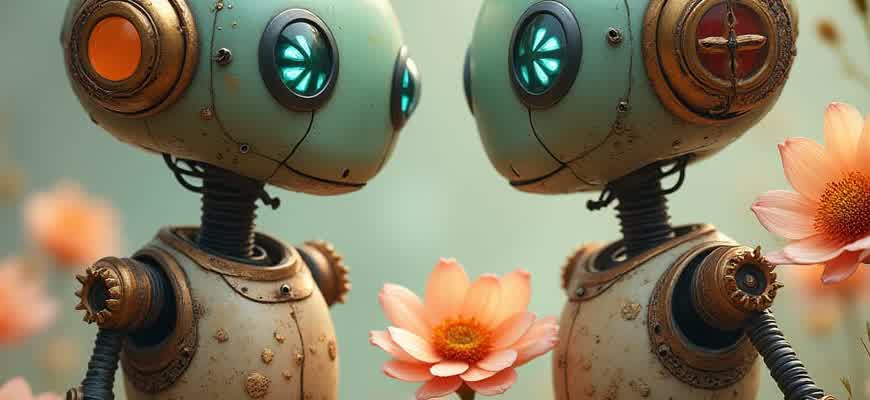
Building Responsive Web Applications
Creating web applications that seamlessly adapt to different screen sizes and devices is a fundamental aspect of modern web development. With the gro...
Read More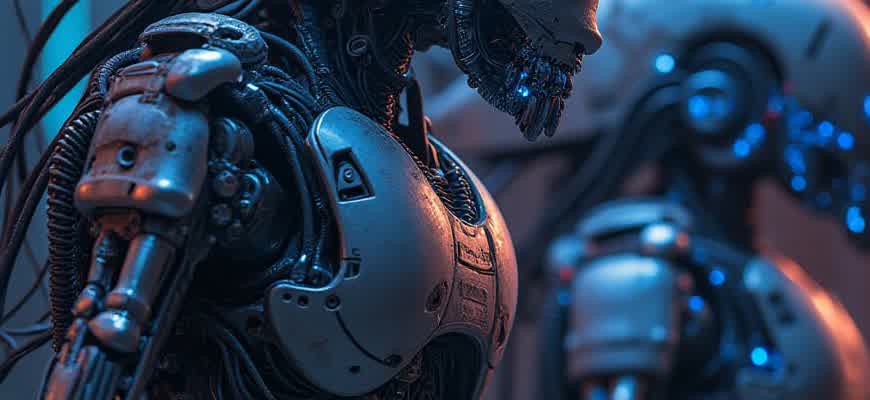
App Development Software for Students
Developing mobile applications has become an essential skill for students interested in technology and design. Several platforms provide a range of f...
Read MoreOur Growth Journey
From humble beginnings to a thriving community of knowledge seekers
100,000+ monthly readers
Launched AI-powered content recommendations, expanded to new languages, and introduced premium membership
75,000+ monthly readers
Introduced video content, launched mobile app, and expanded to new markets
50,000+ monthly readers
Expanded our reach globally, launched new content series, and introduced interactive features
30,000+ monthly readers
Introduced expert interviews, launched our newsletter, and expanded our author network
15,000+ monthly readers
Started our journey with a small team of passionate writers and tech enthusiasts